
On the other hand, all top methods in CAFA2 outperformed their counterparts in CAFA1. NSF DBI-0965768 (ABH). Similarities are computed as Pearsons correlation coefficient between methods, with a 0.75 cutoff for illustration purposes. Nucleic Acids Res. We observe that participating methods usually specialize in one or few categories of protein function prediction, and have been developed with their own application objectives in mind. Genome Biol 17, 184 (2016).
In contrast, the full evaluation mode corresponds to the same type of assessment performed in CAFA1 where all benchmark proteins were used for the evaluation and methods were penalized for not making predictions. Note that here, n Overall, by establishing the state-of-the-art in the field and identifying challenges, CAFA1 set the stage for quantifying progress in the field of protein function prediction over time. Specifically, we calculated the pairwise Pearson correlation between methods on a common set of gene-concept pairs and then visualized these similarities as networks (for BPO, see Fig. The performance improvement from CAFA1 to CAFA2 was calculated as. max=1, which corresponds to the point (1,1) in the precisionrecall plane. Put otherwise, a protein-centric evaluation considers a ranking of ontology terms for a given protein, whereas the term-centric evaluation considers a ranking of protein sequences for a given ontology term. b. CAFA2 benchmark breakdown.
Part of max. The reason for this is that we set the threshold for reporting a discovery when the confidence score for a term was equal to or exceeded the methods F For predicting molecular functions, even though transferring functions from BLAST hits does not give better results, the top models still managed to perform better. Details for all methods are provided in Additional file 1, Precisionrecall curves for top-performing methods. The automated function prediction SIG looks back at 2013 and prepares for 2014. Gapped BLAST and PSI-BLAST: a new generation of protein database search programs. 1 and m e
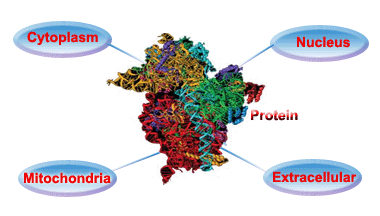
We, thus, collected 357 benchmark proteins for MFO comparisons and 699 for BPO comparisons. volume17, Articlenumber:184 (2016) At the time that authors submitted predictions, we also asked them to select from a list of 30 keywords that best describe their methodology. Bioinformatics. We will refer to the set of all experimentally annotated proteins available at t Automated functional annotation remains an exciting and challenging task, central to understanding genomic data, which are central to biomedical research. max of a method evaluated on the b-th bootstrapped benchmark set. e Three years after CAFA1, the top methods from the community have shown encouraging progress. max scores around 0.6 and considerably surpassed the two baseline models. YM and PNR co-organized the human phenotype challenge. The selection of benchmark proteins for evaluating HPO-term predictors was separated from the GO analyses. However, this effect does not completely explain the extent of the performance improvement achieved by those methods.
For instance, the annotation frequency of organelle (GO:0043226, level 2), intracellular part (GO:0044424, level 3), and cytoplasm (GO:0005737, level 4) are all above the best threshold for the Nave method ( Data The benchmark data and the predictions are available on FigShare https://dx.doi.org/10.6084/m9.figshare.2059944.v1. In total, 56 groups submitting 126 methods participated in CAFA2. In the full evaluation mode n We used evidence codes EXP, IDA, IPI, IMP, IGI, IEP, TAS, and IC to build benchmark and ground-truth sets. This work was a Technology Development effort for ENIGMA Ecosystems and Networks Integrated with Genes and Molecular Assemblies (http://enigma.lbl.gov), a Scientific Focus Area Program at Lawrence Berkeley National Laboratory, which is based upon work supported by the U.S. Department of Energy, Office of Science, Office of Biological & Environmental Research grant DE-AC02-05CH11231. PR, IF, and CSG significantly contributed to writing the manuscript. To assess this term-wise accuracy, we calculated AUCs in the prediction of individual terms. We compared those stored predictions to the newly deposited predictions from CAFA2 on the overlapping set of benchmark proteins and CAFA1 targets. Another possibility is the sparsity of experimental annotations.
PLoS Comput Biol. Clin Pharmacol Ther. In addition to validating investment in the development of new methods, CAFA1 also showed that using machine learning to integrate multiple sequence hits and multiple data types tends to perform well. We compared the results from CAFA1 and CAFA2 using a benchmark set that we created from CAFA1 targets and CAFA2 targets. Ashburner M, Ball CA, Blake JA, Botstein D, Butler H, Cherry JM, et al. It is not very surprising that the top methods achieved good performance for E. coli as it is a well-studied model organism.
For biological process, the other member of the top three is proteinprotein interactions, while for cellular component and molecular function the third member is sequence properties. 11 we show the performance of the top-five methods in predicting the BPO terms that are experimentally verified to be associated with ADAM-TS12. 2013; 29(13):5361. Nat Rev Genet. Predictions for BPO showed a contrasting pattern. Details for all methods are provided in Additional file 1. Both types of evaluation have merits in assessing performance. Proteins without predictions were counted as predictions with a score of 0. While there was methodological improvement between CAFA1 and CAFA2, the interpretation of results and usefulness of individual methods remain context-dependent. At the same time, critically evaluating these tools and understanding the landscape of the function prediction field is a challenging task that extends beyond the capabilities of a single lab. The average performance metric as well as the number of wins were recorded (in the case of identical performance, neither method was awarded a win). Both of these methods were trained on the experimentally annotated proteins available in Swiss-Prot at time t The time line for the second CAFA experiment followed that of the first experiment and is illustrated in Fig. CAFA2 featured expanded analysis compared with CAFA1, with regards to data set size, variety, and assessment metrics. Trends Genet. It is estimated in a maximum likelihood manner as the negative binary logarithm of the conditional probability that the term f is present in a proteins annotation given that all its parent terms are also present. The head-to-head comparisons among the top-five CAFA1 methods against the top-five CAFA2 methods reveal that the top CAFA2 methods outperformed all top CAFA1 methods. Clark WT, Radivojac P. Information-theoretic evaluation of predicted ontological annotations. Not evaluated (organizers) methods are shown in triangles, while benchmark methods (Nave and BLAST) are shown in squares. RPH, MJM, and COD directed the biocuration efforts. EC-U, PD, REF, RH, DL, RCL, MM, ANM, PM-M, KP, and AS performed the biocuration. Solid black lines provide direct is a or part of relationships between terms, while gray lines mark indirect relationships (that is, some terms were not drawn in this picture). max and (2) the numbers in the box indicate the percentage of wins. These metrics and the corresponding evaluation results are shown in Additional file 1. In this particular case, the Paccanaro Lab method did predict the term, but the confidence score was 0.01 below their F In the meantime, more effort will be needed to understand the problems associated with the statistical and computational aspects of method development. The eukarya benchmark rankings therefore coincide with the overall rankings, but the smaller categories typically showed different rankings and may be informative to more specialized research groups. Protein-centric evaluation measures how accurately methods can assign functional terms to a protein. There are complex factors that influence the final ranking including the selection of the ontology, types of benchmark sets and evaluation, as well as evaluation metrics, as discussed earlier. The GOA database: gene ontology annotation updates for 2015. Although various evaluation metrics have been proposed under the framework of multi-label and structured-output learning, the evaluation in this subfield also needs to be interpretable to a broad community of researchers as well as the public. 4, 5, and 6. The total number of leaf terms to predict for biological process was 12; these nodes induced a directed acyclic annotation graph consisting of 89 nodes. CAFA and the open world of protein function predictions. University of Padova grants CPDA138081/13 (ST) and GRIC13AAI9 (EL). Confidence intervals (95 %) were determined using bootstrapping with 10,000 iterations on the set of benchmark sequences. a shows the benchmark sizes for each of the ontologies and compares these numbers to CAFA1. This work was partially supported by the following grants: National Science Foundation grants DBI-1458477 (PR), DBI-1458443 (SDM), DBI-1458390 (CSG), DBI-1458359 (IF), IIS-1319551 (DK), DBI-1262189 (DK), and DBI-1149224 (JC); National Institutes of Health grants R01GM093123 (JC), R01GM097528 (DK), R01GM076990 (PP), R01GM071749 (SEB), R01LM009722 (SDM), and UL1TR000423 (SDM); the National Natural Science Foundation of China grants 3147124 (WT) and 91231116 (WT); the National Basic Research Program of China grant 2012CB316505 (WT); NSERC grant RGPIN 371348-11 (PP); FP7 infrastructure project TransPLANT Award 283496 (ADJvD); Microsoft Research/FAPESP grant 2009/53161-6 and FAPESP fellowship 2010/50491-1 (DCAeS); Biotechnology and Biological Sciences Research Council grants BB/L020505/1 (DTJ), BB/F020481/1 (MJES), BB/K004131/1 (AP), BB/F00964X/1 (AP), and BB/L018241/1 (CD); the Spanish Ministry of Economics and Competitiveness grant BIO2012-40205 (MT); KU Leuven CoE PFV/10/016 SymBioSys (YM); the Newton International Fellowship Scheme of the Royal Society grant NF080750 (TN). The selection of top methods for this study was based on their performance in each ontology on the entire benchmark sets. Article 1] and used for evaluation as the benchmark set. We did not observe any experimental annotation by the time submission was closed. =n, the number of benchmark proteins, whereas in the partial evaluation mode n PubMed Central
3 compare baseline methods trained on different data sets. Wass MN, Mooney SD, Linial M, Radivojac P, Friedberg I. Genome Biology Precision (pr), recall (rc), and the resulting F A document containing a subset of CAFA2 analyses that are equivalent to those provided about the CAFA1 experiment in the CAFA1 supplement. There is also a need to develop an experiment-driven, as opposed to curation-driven, component of the evaluation to address limitations for term-centric evaluation. That is, the way we capture a methods performance in CAFA may not be exactly the same as a user may employ. We originally hypothesized that a possible additional explanation for this effect might be that the average number of HPO terms associated with a human protein is considerably larger than in GO; i.e., the mean number of annotations per protein in HPO is 84, while for MFO, BPO, and CCO, the mean number of annotations per protein is 10, 39, and 14, respectively. To assess this, we analyzed the extent to which methods generated similar predictions within each ontology.
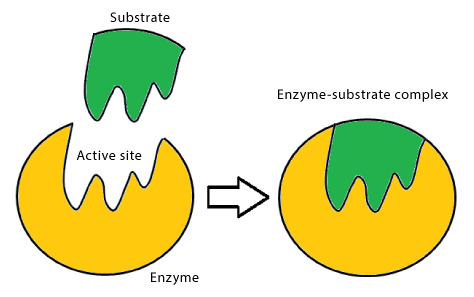