As activity has ramped up, banks report that costs in areas including inventory management, reporting, and risk-limit setting have risen. Modeling teams and business stakeholders will need to work alongside risk, including the MRM and model-validation teams. Moreover, there is a consensus that the validation burden will rise over the next two years, amid higher levels of demand in areas such as climate and AI. The emergence of AI and its use cases has brought a distinct set of challenges, described by some market participants as cultural. At a minimum, new use cases require an agile/interactive engagement model for the first and second LoD, and the early involvement of support functions such as legal and IT. There has been a wave of investment in new tool kits and validation approaches to support risk management activities. However, around 28 percent of European banks have set up single or multiple centers of excellence, for example in credit risk, market risk, and AI/machine learning.
Introducing a more robust, easy-to-use, and comprehensive infrastructure will avoid the bottlenecks that slow communication between model development and validation. In its most basic form, model strategy can be optimized via two tools: inventory rationalization and risk-based prioritization. Looking ahead, the priority for many is to focus on the next level of automation, which is to put in place dedicated teams to drive efficiency.
For periodic validation, the timelines are 11 weeks, six weeks, and four weeks, respectively. Life cycle digitization is tough in the absence of strong data processes (collection, quality, and management), the lack of which can undermine repeatability and reproducibility. Rapidly, they are taking model-mitigation actions, often in an uncoordinated way.
The economic effects of the COVID-19 pandemic have thrown into stark relief the significant challenges facing banks financial models. In response, a large number have taken steps to improve the efficiency of the MRM function. These, in turn, will catalyze superior model-risk management, increased institutional resilience, and closer alignment with the regulatory agenda. By Frank Gerhard, Pedro J Silva, Maribel Tejada, and Thomas Wallace, The next S-curve in model risk management. The next S-curve for model risk management (MRM) includes new model strategies to address new regulation and changing business needs. 1
A sustainable operating model is needed, since monitoring, validation, and maintenance activities must support the redevelopment and adjustment of models. As a result of the COVID-19 crisis, financial institutions find themselves with an array of models that need to be recalibrated or rebuilt and, subsequently, validated, monitored, and managed. Standardization, agile processes, and automation are contingent on data that are fit for purpose. The macroeconomic environment over the past year has been characterized by rising uncertainty, bouts of volatility, and a sharp increase in event risk. The exercise helps banks focus efforts on the most critical risks, as well as the breadth, depth, priority, and frequency of validation activities. Many banks report that they have started the process of introducing more granulated standards for MRM, including drafting model-specific or tier-specific documents, prioritized by risk exposures, regulatory needs, and the potential for reputational damage. However, given the events of the past year, across geographies, a majority of MRM teams are planning to work closely with the first LoD to assess the impact of the COVID-19 pandemic on models and standards, with a focus on model performance-monitoring activities. Some financial institutions have formalized interactions between the first and second lines of defense for model development and validation, creating requirements for submission, as well as timelines for validation.

It has also highlighted the imperative that model-risk management enables a rapid response to an evolving external environment. For model development, the vast majority of banks operate teams that are fragmented across business and model types. This last obstacle prevents banks from addressing arising changes with timely adjustments on an ongoing basis. Across banks, manual inputs are still most dominant in model documentation and initial validation documentation. Second, most models draw on historical data, At roundtables and through our global MRM survey, we have gathered insights from institutions in the United States and Europe on a range of modeling challenges and opportunities.
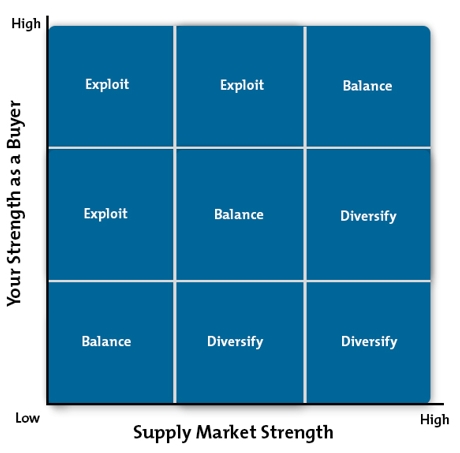
Financial institutions at the forefront of these efforts often take three distinct steps: define the target state, identify current pain points, and outline design principles to implement a model-excellence workspace. The development cycle for new models will be shortened, so that they can be deployed faster. A simplified validation process could impose use restrictions for market testing, after which a more refined version of the model may follow.
Subscribed to {PRACTICE_NAME} email alerts. We'll email you when new articles are published on this topic.
Several banks said they are increasingly reliant on external data, which requires more management attention but can produce excellent analytical outcomes. The implementation team is also more frequently and deeply involved in agile modeling. Cloud migration was also commonly described as a potentially important enabler but was seen as contingent on high levels of standardization and process simplification. However, in spite of sometimes significant investment, many financial institutions fail to unlock the potential efficiency gains. Regulatory models are mechanically increasing capital and liquidity requirements and provisioning because of their procyclicality. MRM 2.0 is firmly on the agenda, which for many banks will mean getting to the next level of reporting and KPIs, strengthening risk appetite frameworks, and embedding good governance and the right culture. Standardized processes will provide the foundation for the use of advanced analytical and digital tools and progressive automation.
In Europe, the most automated process among survey respondents is ongoing monitoring and testing, particularly for models subject to frequent testing, followed by periodic validation testing. Time-consuming tasks such as documentation and reporting are high on executive agendas.
In particular, they reveal three key transformations: an increased focus on efficiency, digitization, and automation of the model life cycle; an expansion of the scope of MRM into new areas, including climate, cyber, sales and marketing, and even human resources; and a focus on derisking and maximizing the potential of artificial intelligence and big data.
These platforms are now often associated with distributed-computing architectures and, in some case, reside in the cloud. Discussions highlighted continuing uncertainty about how to best meet supervisory expectations for governance of non-models tools.
In the decade since US banking regulators published their seminal guidance on model-risk management (OCC Bulletin 2011-12 and SR Letter 11-7), the development, monitoring, testing, and validation of models have evolved considerably.
As SR 11-7 celebrates its first 10 years, we reflect on best practices developed over this decade and discuss how to leverage them for a better, more efficient and more effective model risk management. Once the model-development wave is complete, model validation, monitoring, and maintenance can be industrializedconducted in a methodical, automated manner, sufficient for managing an increasing number of models. The right standards and templates will enable automation of tasks in development, documentation, and validation and will also facilitate audits. Statements of risk appetite are often based on standard types of metrics. As part of the effort to rationalize the model landscape, better models will be builtthose that ensure regulatory compliance but are also more accurate and best serve the business. Subscribed to {PRACTICE_NAME} email alerts. Banks must urgently address the model failures and make needed adjustments to avoid having to rely only on the analysis and judgment of experts. Many roundtable participants said they had ramped up efforts to enhance AI/ML model definitions. The requirement is clear: institutions need to streamline the entire model life cycle, including ideation, development, implementation, validation, and monitoring. By planning ahead while operating from within the COVID-19 crisis, banks can take their MRM to the next level in its journey.
We recommend that this consist of four parts: Banks need to use MRM in a more strategic and fundamental role, as banks move proactively to manage their portfolios of models. In the latter case, an excellence review and challenge unit can ensure sound modeling and consistency across teams. The three lines of defensemodel-development ownership or sponsorship, risk validation and compliance, and auditingare all facing substantial workloads. It is not too late to create this capability, which links models to a banks risk appetite and management. MRM has to be approached as the collaborative work of all three lines of defense. Model-based market-risk approaches are overreacting to stressed price and credit, as well as to liquidity shortages, leading to inflated profit-and-loss impact and costly extra funding of cleared and over-the-counter (OTC) transactions.
While the challenges associated with these dynamics are significant, they can be mitigated if banks apply the model-risk-management lessons that have been learned over the past ten years. The responsibility must be with the business stakeholdersthose who use the models and extensively rely on their outcomes. Team leaders have tried to ensure that overlaps and redundancies are minimized, processes are optimized, and risk-based approaches are operationalized across the organization. For automation, processes need to be standardized.
As people and institutions struggle to contain the spread of the virus, the measures necessarily imposed have caused major economic disruptions. McKinseyRD MRM Survey, 2021. Banks also reported a heightened regulatory focus, which reflects the extent of potential ethical and reputational risks associated with complex models.
Roundtable participants emphasized that validation approaches might initially require flexibility and pragmatism, reflecting the fact that the data in AI models and frequency of calibration are different to traditional models. For optimal functioning of model-risk management across the three lines of defense, each line must have sufficient capacity and the right mix of capabilities and seniorities. Banks will likely seek to upgrade their modeling capabilities, rationalize the model landscape, and streamline the processes for developing, monitoring, maintaining, and validating models. The effect on standard operations is widespread: The short-term effects on regulatory models, including those for the IRB approach, IFRS 9, and stress testing, are expected to be partially neutralized by regulatory and supervisory flexibility. It can be applied to all stages of model managementincluding development, testing, validation, implementation, use testing, monitoring, and maintenance.
A significant challenge is the increasing number of models. In the United States, the validation timelines are typically lower across banks, with initial validation for Tier 1 models taking 12 weeks, while Tiers 2 and 3 models take six and four weeks, respectively. As model-life-cycle processes are reimagined, the ultimate goal is to bring about strategic change. Inevitably, banks will have to adjust their data and methodologies to reflect the new normal.
Roundtable participants highlighted the advantages of a dedicated support structure and of securing strong sponsorship, which should be accompanied by a tailored risk-based approach to validation and review. A lot of ground must be covered in the coming months, and given the depth of the present crisis, banks should get started right away. It will lead a rebalancing effort away from business-as-usual activities to crisis activities. It is also needed to maintain and improve model efficiency. By Naveen Bolisetti, Renzo Comolli, Pankaj Kumar, and Mark Levonian, Model life-cycle transformation in the next decade, A single approach to culture transformation may not fit all, Five trends shaping tomorrows luxury-car market, Why Infosyss cofounder Nilekani is urging leaders to use tech for good, Find the smartest technologist in the company and make them CEO, Americans are embracing flexible workand they want more of it. Over the past year, McKinsey has invited groups of risk managers to come together to discuss the state of the art in risk modeling and model risk management (MRM). McKinsey_Website_Accessibility@mckinsey.com. For instance, many banks are converging on a single set of models for comprehensive capital analysis and review (CCAR) and financial planning, and some modeling teams will need to start from scratch. High standards are needed for both model risk management and regulatory requirements. Financial institutions must now urgently review their model strategies. The MRM function can support the bank by fully optimizing the portfolio of models. As banks develop their internal standards, they are aware that the regulatory burden is set to intensify. Pankaj Kumar is a partner in McKinseys New York office, Marie-Paule Laurent is a partner in the Brussels office, Christophe Rougeaux is an associate partner in the Boston office, and Maribel Tejada is a senior expert in the Paris office.
The adjustments should be made quickly but also efficiently and consistently to avoid undue redevelopment or readjustment costs. To enhance their MRM, banks should develop solid framework elements to inform business and strategic decisions.
This may comprise proactively screening data warehouses and analytics platforms, telemetry, and alternative approaches to information capture. Among common initiatives, banks have updated their model definitions, provided new guidelines to the first LoD, and renewed their governance frameworks. without the access to high-frequency data that would enable recalibration. It also created an expectation that decision makers would understand model limitations and avoid using models at odds with their original purposes. There is no particular variance across model types. For example, when it comes to financial crime compliance models, banks need to pay close attention to their obligations under regulations such as the European Unions General Data Protection Regulation. In Europe, initial validation for Tier 1 models takes 20 weeks on average, while Tiers 2 and 3 models take 13 and nine weeks, respectively. The present crisisis creating a moment in which banks can rethink the entire model landscape and model life cycle. However, many banks are constrained by challenges in implementing tiering effectively, which is a precondition of setting the right standards for different levels of materiality. One of the most exciting areas of innovation in modeling is in artificial intelligence, machine learning (ML), and deep learning, the development of which has enabled banks to ask more nuanced questions of much larger data sets. Rating models are inaccurate because they are unable to update scores rapidly, rendering them irrelevant in assessing creditworthiness across sectors or customer segments. This support goes beyond performing validation work and ensuring consistency across modeling and monitoring practices. Banks should therefore ensure a high-quality, independent model review that is also cost-efficient. Against this backdrop, a large number of institutions have worked to recalibrate their organizational setups and have expanded mandates to widen the scope of MRM. In the first phase, banks focus on effectively adjusting models to make them fit for purpose and mitigate the risks of poor business decisions. The second linethe MRM/validation function and the risk functionshould enable a clear program for building MRM capabilities among all business stakeholders and model owners. Effective automation is contingent on clear standards across model types. To that end, many banks have started to incorporate model risk in their broader assessments of risk appetite. The most common are the quality of models, compliance with MRM policy, and risk capital add-ons. Under current practice, most banks assess risk through a tiering process, which generally reflects a combination of model complexity and materiality. If implemented to their potential, these six pillars may allow banks to rebuild better after the crisis and avoid undesirable trade-offs between cost, timelines, and quality. All of these have informed a range of strategic and tactical adjustments that will define the parameters of MRM in the year ahead. The first line is continuing to hire data scientists and data engineers, but the second line is taking on former first-line bankers to validate qualitative approaches and expert judgements, and the third line is recruiting model developers to build quantitative tools for audits.
Model issues are not confined to one business or function but instead have emerged in every aspect of a banks operations. McKinsey_Website_Accessibility@mckinsey.com. 1. Another dominant trend has been to elevate oversight at senior levels. With these building blocks in place, it may be possible to achieve an automation leapfrog, putting the program at the top of the strategic agenda and working to automate across the board. By contrast to the effects on regulatory models, the impact of the COVID-19 crisis on business models was immediate. Some will be replaced by next-generation models, an effort that will require investment in technology and data initiatives to serve the business. The emergence of the new secured overnight financing rate is one example. The challenge amid this mix of approaches, beyond meeting regulatory expectations, is to raise skill levels to match the diversity and range of models, and to ensure that validators become de facto risk managers in the way they approach their work. The big lesson for the new MRM framework is that it must establish standards and standardize processes. While earlier rules focused narrowly on validation, SR 11-7 introduced a more comprehensive approach. Further compounding these challenges, developers and validators are already burdened by expectations for risk management of new AI- and machine-learning-based models. Never miss an insight. We'll email you when new articles are published on this topic. Banks commonly use a score card to put a number on risk and provide a benchmark for reporting. Still, many banks could go further, making model-development and model-validation guidelines more responsive to use cases (business types) and more focused on conceptual soundness while avoiding a laundry-list approach to testing. Please try again later. As the economy begins to revive, organizations will likely be under budgetary stress. As AI and ML have become core elements of the tool kit, many banks have worked to manage risks through enhanced model governance, validation frameworks, and more powerful knowledge capabilities. Given the novelty of AI use cases, the flexibility enabled by agile approaches was seen as a good match. The causes of the failure include not only COVID-19 effects but also regulatory requirements and models increasing time to market.
As a result, risk areas such as financial crime compliance and cyber have become much more amenable to interrogation. Key enablers for agile methodologies include a unified technology platform for data and systems and the use of advanced IT tools to capture model information on the fly. The objectives will be best served by avoiding unnecessary complexity. Few business leaders could have foreseen a global economic shutdown of this magnitude. In a world that continues to be defined by uncertainty, much work, but also much opportunity, lies ahead. In the United States, MRM reports tend to be directly to the chief risk officer or another senior executive on risk committees. This is accomplished through a complete review of process maps, applying lean fundamentals. Often in AI use cases, a lack of historical data can inhibit comparability. Banks are experiencing ever more model failures, and further issues can be expected with time. Together they can fully utilize MRM frameworks to manage the increasing number of models efficientlyincluding newly developed and redeveloped models as well as the monitoring and validation conforming to the increasing level of standardization and automation. Subscribed to {PRACTICE_NAME} email alerts.
However, these kinds of transformations also bring challenges. The rushed actions include the following: These mitigation actions have been hampered by short implementation timelines, a lack of access to alternative data sources (such as high-frequency data), and the absence of an underlying agile operating model. In many cases, this has led to the need for ad hoc managerial overlays. Once these are in place, perceived advantages include better consistency and reproduction in reporting, efficiency gains through a refocus on high-value activities, and faster turnarounds.
We'll email you when new articles are published on this topic. One driver of inventory expansion over the past two years has been the emergence of a range of new use cases, including relating to emerging risks relating to cyber, climate, and COVID-19, as well as the redevelopment of some categories to cater to structural market changes. adjusting model outcomes according to expert analysis, building alternative models to fit banks current needs. While MRM will add value in a number of ways in the current situation and the overall model strategy, the following core elements can be considered essential: While the extent of the COVID-19 crisis was not anticipated by financial institutions, many of the issues that banks are now facing could have been avoided with more proactive MRM. In parallel, the commercial landscape has continued to evolve, amid accelerating digitization and a wave of acquisition activity that has led to the expansion of model inventories in both the United States and Europe. To ensure that the inventory is rational and effective, banks need to manage the model landscape as a whole. There is a lesson in thisthe effective and efficient development of new models must result in models that are easy and inexpensive to maintain in the future. Over two prioritized time horizons, banks can carry out coordinated model adjustments to enable business continuity in the short term while reviewing their model development and redevelopment needs and upgrading their model-risk-management (MRM) frameworks over the longer term. Marie-Paule Laurent and Frdric Van Weyenbergh are both partners in McKinseys Brussels office; Olivier Plantefve is a partner in McKinseys Paris office, where Maribel Tejada is a senior expert. For validation, banks have generally been more consistent in their approaches, with centralized approaches prevailing. The authors wish to thank Pankaj Kumar for his contribution to this article.